4.1 Neural Networks
Contents
4.1 Neural Networks#
A linear regression is a single, fully connected neuron.
Figure: Extracted from “Hands on Maching Learning using Keras and Tensorflow”
The simplest neural network is a logistic regression
\(y = f( \sum_{i=1}^3 (w_i x_i + b_i))\),
where \(y\) is the output, \(w_i\), are the weights, \(b_i\) are the biases in the neuron, and \(f\) is an activation function (e.g., sigmoid, ReLu, etc)
For classification problem, it is called a Threshold Logic Unit TLU because it outputs a linear combination of the inputs, and if the result exceeds a threshold, it outputs the positive class.
A perceptron is a single layer of TLUs, which each TLU connected to all the inputs.
Logistic regression: It is just a one layer neural network classifier
We will explore the classification problem with NN.
import torch
import torch.nn as nn
import torch.optim as optim
import matplotlib.pyplot as plt
import numpy as np
1. Dataset in Pytorch#
We will read a dataset and convert into a format readable by PyTorch.
We have a dataset of images of digits (0 to 9). Each image is made of 8 * 8 pixels in grey scale. We start from the familiar sklearn
digits data set, which provides images as arrays and labels as integers.
The data is first cast as an array and we will prepare it as a PyTorch ready data.
1.1 Load arrays#
# load data set in memory
from sklearn.datasets import load_digits
digits = load_digits() # load data set
data,labels = digits["data"].copy(),digits["target"].copy() # copy data and target
data = data.astype(np.float32).reshape(-1,8,8) # reshape data on 8x8 grid
print(data.shape,labels.shape)
(1797, 8, 8) (1797,)
The data set has 1797 8 x 8 images.
Now that we have data and labels, we have to prepare the data sets so that it can be used in PyTorch. The main steps are:
Wrap these into a Pytorch
DataSet
, which will also convert the arrays intotensors
.Split between training and testing data using
random_split
pytorch functionThen load the datasets with
DataLoader
1.2 Create a custom dataset class#
PyTorch provides the Dataset
class that you can use to create a custom dataset. You need to implement the len and getitem methods.
from torch.utils.data import Dataset
class CustomDataset(Dataset): # create custom dataset
def __init__(self, data,labels): # initialize
self.data = data
self.labels = labels
def __len__(self):
return len(self.data)
def __getitem__(self, index):
sample_data = self.data[index]
sample_labels = self.labels[index]
return torch.Tensor(sample_data),(sample_labels) # return data as a tensor
custom_dataset = CustomDataset(data, labels)
# Example iteration through the training DataLoader
for batch_data, batch_labels in custom_dataset:
# Your training loop here
print("Train Batch Data:", batch_data)
print("Train Batch Labels:", batch_labels)
break
Train Batch Data: tensor([[ 0., 0., 5., 13., 9., 1., 0., 0.],
[ 0., 0., 13., 15., 10., 15., 5., 0.],
[ 0., 3., 15., 2., 0., 11., 8., 0.],
[ 0., 4., 12., 0., 0., 8., 8., 0.],
[ 0., 5., 8., 0., 0., 9., 8., 0.],
[ 0., 4., 11., 0., 1., 12., 7., 0.],
[ 0., 2., 14., 5., 10., 12., 0., 0.],
[ 0., 0., 6., 13., 10., 0., 0., 0.]])
Train Batch Labels: 0
1.3 Random split training and testing#
from torch.utils.data import random_split
# Determine the size of the training set
train_size = int(0.8 * len(custom_dataset)) # 80% of the data set
test_size = len(custom_dataset) - train_size # 20% of the data set
# Use random_split to create training and testing subsets
train_dataset, test_dataset = random_split(custom_dataset, [train_size, test_size])
1.4 Create a DataLoader#
Once you have your custom dataset, you can use a DataLoader to handle batching, shuffling, and other data loading functionalities.
from torch.utils.data import DataLoader
# Create a DataLoader
batch_size = 32 # Adjust according to your needs
shuffle = True # Set to True if you want to shuffle the data
data_loader_train = DataLoader(train_dataset, batch_size=batch_size, shuffle=shuffle)
data_loader_test = DataLoader(test_dataset, batch_size=batch_size, shuffle=shuffle)
# Example iteration through the testing DataLoader
for batch_data, batch_labels in data_loader_test:
# Your testing loop here
print("Test Batch Data:", batch_data.shape)
print("Test Batch Labels:", batch_labels.shape)
break
Test Batch Data: torch.Size([32, 8, 8])
Test Batch Labels: torch.Size([32])
2. Design Model#
The first neural networks we will create is a single neuron that takes the images as input and output the probability in each of the 10 classes.
# We create a subclass of neural networks:
# #This one will just have one layer
class NN1(nn.Module): # NN1 inherits from nn.Module
# this defines the arcitecture of the NN
def __init__(self, size_img, num_classes):
# Here we define all the functions that we will use during the forward part (data -> prediction)
# super means that the model will inherit all of the methods
super(NN1, self).__init__()
self.flatten = nn.Flatten() # go from a 8*8 tensor to a 64*1 tensor
self.size_img = size_img # number of pixels in an image
self.layer1 = nn.Linear(size_img, num_classes) # y = wx + b with w = 10*64 (10 digits * 256 pixels)
# this defines how the data passes through the layers
def forward(self, x):
x = self.flatten(x) # go from a 8*8 tensor to a 64*1 tensor
logits = self.layer1(x) # y = wx + b with w = 10*64 (10 digits * 256 pixels)
return torch.sigmoid(logits) # apply sigmoid function to the output of the layer to get a probability
We now create a model of the class NN1.
model = NN1(8*8, 10)
print(model)
NN1(
(flatten): Flatten(start_dim=1, end_dim=-1)
(layer1): Linear(in_features=64, out_features=10, bias=True)
)
3. Loss Function#
For the binary classification, we had defined the logistic function or sigmoid function as,
\(\hat{y} = \frac{1}{1 + e^{-z}}\),
as the probability function for a binary classifier.
The log loss function to minimize is:
\(\mathcal{L} = - (y \log \hat{y} + (1 - y) \log (1 - \hat{y})\) with \(\hat{y} = \frac{1}{1 + e^{-z}}\).
We wanted to find all of the weights \(w\) and biases \(b\) such that \(P(Y = y)\) is maximum, which is equivalent to minimizing the loss \(\mathcal{L}\).
For a multi-class classification, \(k\) being the class and \(K\) the number of classes, the sigmoid function is extended to a softmax function,
\(P(Y = k) = \frac{\exp(w_k x + b_k)}{\sum_{j = 1}^K \exp(w_j x + b_j)}\)
Once we have found the values of the \(w_k\) and \(b_k\) for \(k = 1 , \cdots , K\), we can compute the values of the \(P(Y = k)\). We then look for which value of \(k\) \(P(Y = k)\) is maximal and we classify this sample as class \(k\).
Similarly, we define the loss with \(K\) classes:
\(\mathcal{L} = - \sum_{k = 1}^K y_k \log \frac{\exp(w_k x + b_k)}{\sum_{j = 1}^K \exp(w_j x + b_j)}\). We have \(y_k = 1\) if the true label associated with the sample \(x\) is \(k\), otherwise \(y_k = 0\).
This is called the cross-entropy loss.
nn.CrossEntropyLoss()
CrossEntropyLoss()
4. Optimization#
Gradient descent uses the entire data set to compute the gradient and find the optimal (minimum loss) solution. Effectively, the batch size is the entire data set.
Mini batch gradient descent uses batches of data to compute the gradient, find a solution, and moves to the next set of training data. The batch size is determined. The prediction on the training set is calculated for each sample in the batch, then averaged. Then the gradient is calculated.
Stochastic gradient descent uses one data sample to calculate the gradient, finds a solution, then take another training sample to go down the gradient. Effectively, the batch size is 1.
In this example, we will use stochastic gradient descent SGD.
Parameters to choose are:
optimizer (gradient descent or others)
learning rate (scale to jump with the gradient)
learning_rate=0.001
optimizer = optim.SGD(model.parameters(), lr=learning_rate)
5. Training#
Parameters to choose are:
batch size: this is the size of an individual training set used to estimate an average model prediction loss, after which a gradient will be calculated, and the model weights updated.
number of epochs: this is the number of times the training goes over the entire data sets. If the training set is split into batches of size
batch_size
, then at each epoch, the training will be performed over all batches. Models tend to be trained over multiple-to-many epochs (10,100,1000).
Let us now define how to train the model. We will create a function for that.
def train(model, n_epochs, trainloader, testloader=None,learning_rate=0.001 ):
# Define loss and optimization method
criterion = nn.CrossEntropyLoss()
optimizer = optim.SGD(model.parameters(), lr=learning_rate)
# # Save loss and error for plotting
loss_time = np.zeros(n_epochs)
accuracy_time = np.zeros(n_epochs)
# Loop on number of epochs
for epoch in range(n_epochs):
# Initialize the loss
running_loss = 0
# Loop on samples in train set
for data in trainloader:
# Get the sample and modify the format for PyTorch
inputs, labels = data[0], data[1]
inputs = inputs.float()
labels = labels.long()
# Set the parameter gradients to zero
optimizer.zero_grad()
outputs = model(inputs)
loss = criterion(outputs, labels)
# Propagate the loss backward
loss.backward()
# Update the gradients
optimizer.step()
# Add the value of the loss for this sample
running_loss += loss.item()
# Save loss at the end of each epoch
loss_time[epoch] = running_loss/len(trainloader)
# After each epoch, evaluate the performance on the test set
if testloader is not None:
correct = 0
total = 0
# We evaluate the model, so we do not need the gradient
with torch.no_grad(): # Context-manager that disabled gradient calculation.
# Loop on samples in test set
for data in testloader:
# Get the sample and modify the format for PyTorch
inputs, labels = data[0], data[1]
inputs = inputs.float()
labels = labels.long()
# Use model for sample in the test set
outputs = model(inputs)
# Compare predicted label and true label
_, predicted = torch.max(outputs.data, 1)
total += labels.size(0)
correct += (predicted == labels).sum().item()
# Save error at the end of each epochs
accuracy_time[epoch] = 100 * correct / total
# Print intermediate results on screen
if testloader is not None:
print('[Epoch %d] loss: %.3f - accuracy: %.3f' %
(epoch + 1, running_loss/len(trainloader), 100 * correct / total))
else:
print('[Epoch %d] loss: %.3f' %
(epoch + 1, running_loss/len(trainloader)))
# Save history of loss and test error
if testloader is not None:
return (loss_time, accuracy_time)
else:
return (loss_time)
# (loss, error) = train(model, 10,trainloader, testloader, learning_rate)
(loss, accuracy) = train(model, 100,data_loader_train, data_loader_test)
[Epoch 1] loss: 2.332 - accuracy: 18.333
[Epoch 2] loss: 2.302 - accuracy: 19.444
[Epoch 3] loss: 2.273 - accuracy: 19.167
[Epoch 4] loss: 2.245 - accuracy: 19.722
[Epoch 5] loss: 2.214 - accuracy: 17.500
[Epoch 6] loss: 2.182 - accuracy: 16.944
[Epoch 7] loss: 2.153 - accuracy: 19.167
[Epoch 8] loss: 2.126 - accuracy: 21.944
[Epoch 9] loss: 2.102 - accuracy: 23.611
[Epoch 10] loss: 2.080 - accuracy: 25.278
[Epoch 11] loss: 2.059 - accuracy: 30.000
[Epoch 12] loss: 2.039 - accuracy: 33.056
[Epoch 13] loss: 2.021 - accuracy: 36.667
[Epoch 14] loss: 2.004 - accuracy: 40.000
[Epoch 15] loss: 1.988 - accuracy: 42.222
[Epoch 16] loss: 1.973 - accuracy: 45.278
[Epoch 17] loss: 1.959 - accuracy: 47.778
[Epoch 18] loss: 1.946 - accuracy: 50.000
[Epoch 19] loss: 1.934 - accuracy: 53.056
[Epoch 20] loss: 1.923 - accuracy: 55.556
[Epoch 21] loss: 1.912 - accuracy: 57.778
[Epoch 22] loss: 1.903 - accuracy: 59.722
[Epoch 23] loss: 1.894 - accuracy: 62.222
[Epoch 24] loss: 1.886 - accuracy: 63.333
[Epoch 25] loss: 1.878 - accuracy: 64.722
[Epoch 26] loss: 1.871 - accuracy: 65.278
[Epoch 27] loss: 1.864 - accuracy: 66.111
[Epoch 28] loss: 1.857 - accuracy: 66.667
[Epoch 29] loss: 1.851 - accuracy: 67.500
[Epoch 30] loss: 1.846 - accuracy: 68.333
[Epoch 31] loss: 1.841 - accuracy: 68.889
[Epoch 32] loss: 1.836 - accuracy: 69.167
[Epoch 33] loss: 1.831 - accuracy: 70.000
[Epoch 34] loss: 1.827 - accuracy: 70.278
[Epoch 35] loss: 1.823 - accuracy: 70.833
[Epoch 36] loss: 1.819 - accuracy: 70.833
[Epoch 37] loss: 1.815 - accuracy: 70.833
[Epoch 38] loss: 1.812 - accuracy: 70.833
[Epoch 39] loss: 1.808 - accuracy: 70.833
[Epoch 40] loss: 1.805 - accuracy: 70.833
[Epoch 41] loss: 1.802 - accuracy: 71.111
[Epoch 42] loss: 1.799 - accuracy: 71.389
[Epoch 43] loss: 1.796 - accuracy: 71.944
[Epoch 44] loss: 1.794 - accuracy: 72.778
[Epoch 45] loss: 1.791 - accuracy: 72.778
[Epoch 46] loss: 1.789 - accuracy: 72.778
[Epoch 47] loss: 1.787 - accuracy: 72.778
[Epoch 48] loss: 1.784 - accuracy: 72.778
[Epoch 49] loss: 1.782 - accuracy: 72.778
[Epoch 50] loss: 1.780 - accuracy: 72.778
[Epoch 51] loss: 1.778 - accuracy: 73.056
[Epoch 52] loss: 1.776 - accuracy: 73.056
[Epoch 53] loss: 1.774 - accuracy: 73.333
[Epoch 54] loss: 1.773 - accuracy: 73.333
[Epoch 55] loss: 1.771 - accuracy: 73.611
[Epoch 56] loss: 1.769 - accuracy: 73.889
[Epoch 57] loss: 1.768 - accuracy: 74.167
[Epoch 58] loss: 1.766 - accuracy: 74.444
[Epoch 59] loss: 1.765 - accuracy: 74.722
[Epoch 60] loss: 1.763 - accuracy: 74.722
[Epoch 61] loss: 1.762 - accuracy: 75.000
[Epoch 62] loss: 1.761 - accuracy: 75.278
[Epoch 63] loss: 1.759 - accuracy: 75.556
[Epoch 64] loss: 1.758 - accuracy: 75.833
[Epoch 65] loss: 1.757 - accuracy: 76.111
[Epoch 66] loss: 1.755 - accuracy: 76.667
[Epoch 67] loss: 1.754 - accuracy: 76.389
[Epoch 68] loss: 1.753 - accuracy: 76.667
[Epoch 69] loss: 1.752 - accuracy: 76.667
[Epoch 70] loss: 1.751 - accuracy: 76.944
[Epoch 71] loss: 1.750 - accuracy: 77.222
[Epoch 72] loss: 1.749 - accuracy: 77.222
[Epoch 73] loss: 1.748 - accuracy: 77.222
[Epoch 74] loss: 1.747 - accuracy: 77.222
[Epoch 75] loss: 1.746 - accuracy: 77.222
[Epoch 76] loss: 1.745 - accuracy: 77.222
[Epoch 77] loss: 1.744 - accuracy: 77.222
[Epoch 78] loss: 1.744 - accuracy: 77.222
[Epoch 79] loss: 1.743 - accuracy: 77.222
[Epoch 80] loss: 1.741 - accuracy: 77.222
[Epoch 81] loss: 1.741 - accuracy: 76.944
[Epoch 82] loss: 1.740 - accuracy: 76.944
[Epoch 83] loss: 1.739 - accuracy: 76.944
[Epoch 84] loss: 1.738 - accuracy: 76.944
[Epoch 85] loss: 1.738 - accuracy: 76.944
[Epoch 86] loss: 1.737 - accuracy: 76.944
[Epoch 87] loss: 1.736 - accuracy: 76.944
[Epoch 88] loss: 1.735 - accuracy: 76.944
[Epoch 89] loss: 1.735 - accuracy: 76.944
[Epoch 90] loss: 1.734 - accuracy: 76.944
[Epoch 91] loss: 1.733 - accuracy: 76.944
[Epoch 92] loss: 1.733 - accuracy: 76.944
[Epoch 93] loss: 1.732 - accuracy: 76.944
[Epoch 94] loss: 1.732 - accuracy: 76.944
[Epoch 95] loss: 1.731 - accuracy: 77.222
[Epoch 96] loss: 1.730 - accuracy: 77.222
[Epoch 97] loss: 1.730 - accuracy: 77.222
[Epoch 98] loss: 1.729 - accuracy: 77.222
[Epoch 99] loss: 1.729 - accuracy: 77.222
[Epoch 100] loss: 1.728 - accuracy: 77.222
Let us now plot the evolution of the loss and the percentage of correct predictions with time:
fig, ax1 = plt.subplots()
color = 'tab:red'
ax1.set_xlabel('Epoch')
ax1.set_ylabel('Loss', color=color)
ax1.plot(np.arange(1, 101), loss, color=color)
ax1.tick_params(axis='y', labelcolor=color)
ax2 = ax1.twinx()
color = 'tab:blue'
ax2.set_ylabel('Correct predictions', color=color)
ax2.plot(np.arange(1, 101), accuracy, color=color)
ax2.tick_params(axis='y', labelcolor=color)
fig.tight_layout()
plt.show()
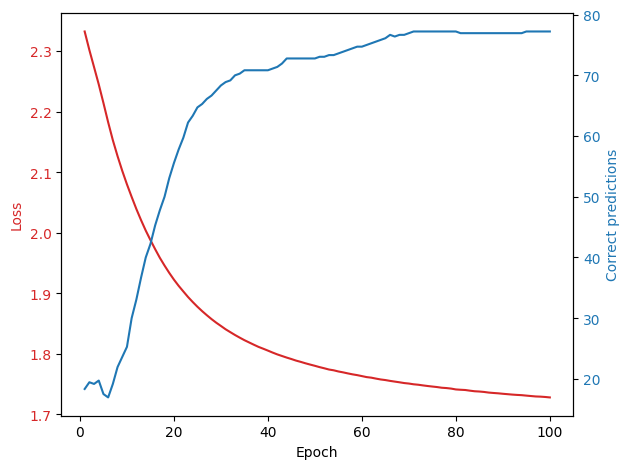